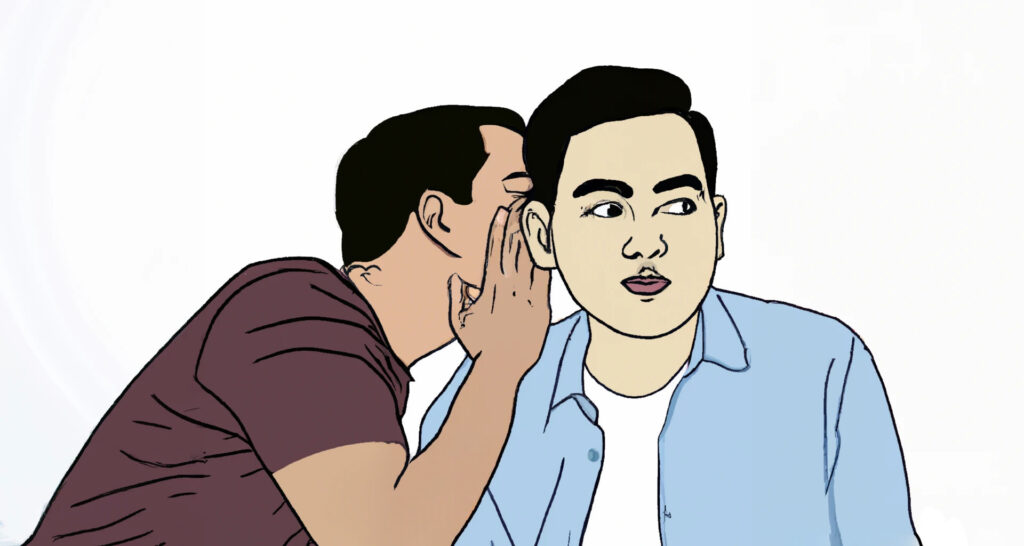
Yesterday OpenAI published a new paper about multilingual speech recognition and, to everyone’s surprise, they also publicly released the model. Thanks to a mix of curiosity and procrastination, I tried it out in Polish on various domains such as slang, politics and medical text books.
Simple language
Let’s start with something simple.
Input/ground truth 🇵🇱 :
“Poszedłem do sklepu kupić pomidory, ale ich nie było, więc kupiłem ogórki. Przy kasie zauważyłem, że są gumy do żucia, więc też je wziąłem. W końcu wróciłem do domu i zjadłem śniadanie.”
English translation 🇬🇧:
“I went to the store to buy tomatoes, but they were gone, so I bought cucumbers. At checkout I noticed there were chewing gums so I took them too. Eventually I came home and ate breakfast.”
Whisper 🗣:
“Poszedłem do sklepu kupić pomidory, ale ich nie było, więc kupiłem ogórki. Przy kasie zauważyłem, że są gumę dorzucia, więc też się wdziąłem. W końcu wróciłem do domu i zjadłem śnalenia.”
Apart from 3 mistakes, the transcription is correct. Whisper has merged “do żucia” into “dorzucia” which sounds the same, but is not an actual word. It also made up the word “śnalenia” instead of “śniadania”.
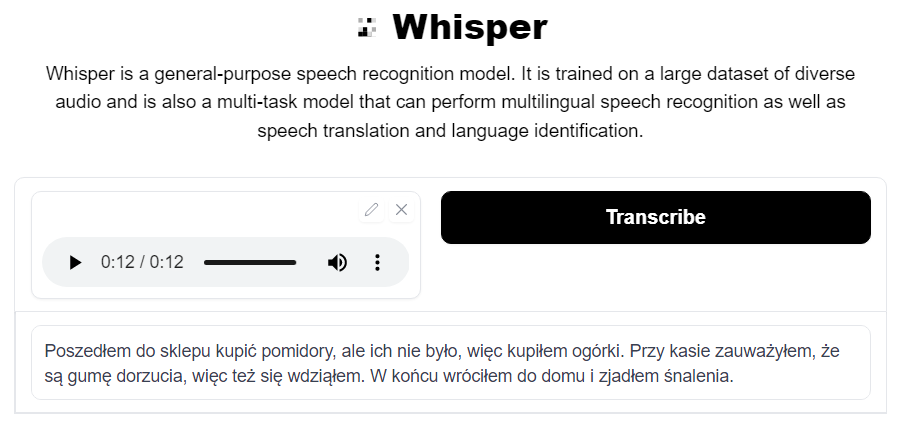
News
Input/ground truth 🇵🇱 :
“W tej chwili trzeba palić wszystkim poza oponami czy podobnie szkodliwym rzeczami, bo Polska musi być ogrzana i rzeczywiście śmieciami niektórzy zaczęli palić. A skoro o śmieciach mowa, to wrócimy też do słów Władimira Putina.”
English translation 🇬🇧:
“At the moment you have to burn everything except tires or similar harmful things, because Poland needs to be heated and some people have started to burn rubbish. And since we are talking about rubbish, we will also return to the words of Vladimir Putin.“
Whisper 🗣:
“W tej chwili trzeba palić wszystkim, poza oponami czy podobnie szkodliwimy rzeczami, bo Polska musi być ogrzana i rzeczywiście śmieciami niektóry zaczęli palić. Skoro jest śmieciach mowa, to wrócimy też do stółu Wadimira Pudina.”
This example has a similar outcome. Replacement of two similar letters from “szkodliwymi” to “szkodliwimy”, “słów” became “stółu” and “Władimira Putina” (Vladimir Putin) turned into “Wadimira Pudina”.
Source: https://www.youtube.com/watch?v=l49bYznR-og
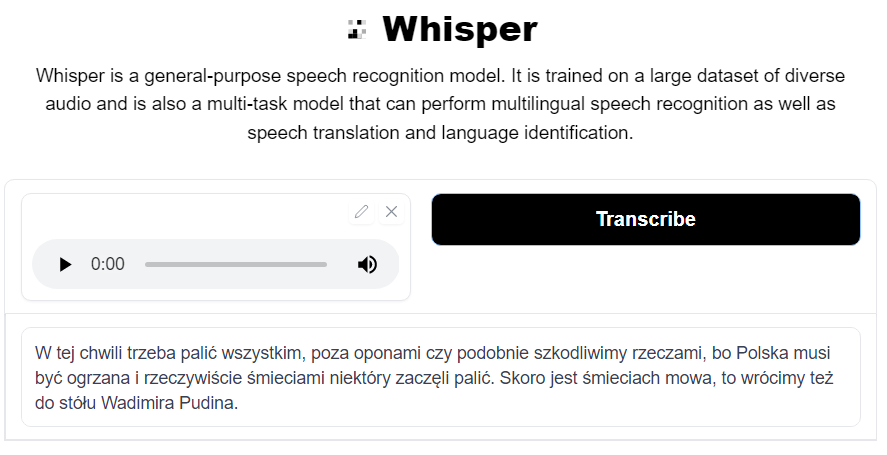
Parliament proceedings
Input/ground truth 🇵🇱 :
“Szanowny Panie Marszałku, Szanowny Panie Ministrze, Wysoki Sejmie. Mam zaszczyt przedstawić sprawozdanie z druku 2475, Komisji Gospodarki i Rozwoju oraz Komisji Spraw Zagranicznych o rządowym projekcie ustawy o ratyfikacji porozumienia między Rzeczpospolitą Polską a Republiką Austrii o wygaśnięciu skutków prawnych artykułu 11, ustęp 3.”
English translation 🇬🇧:
“Dear Speaker of the Parliament, Dear Mr. Minister, Honorable Parlament. I have the honor to present the report from the 2475 press, the Committee on Economy and Development and the Committee on Foreign Affairs on the government bill on the ratification of the agreement between the Republic of Poland and the Republic of Austria on the expiry of the legal effects of article 11, paragraph 3.“
Whisper 🗣:
“Szanowny Panie Marszałku, Szanowny Panie Ministrze Wiosoki Sejmie, mamy zaszczyt przedstawić spraw zdanie z druku 2475 Komisji Gospodarki i Rozwoju oraz Komisji Spraw Zagranicznych o rządowym projekcie ustawy, o ratyfikacji porozumienia między Rzeczpospolitaną Polską a Republiką Austrii, o wygaśnięciu skutków prawnych artykułu 11 ustęp 3.”
This extract is as formal as it gets, similar to legal documents etc. As far as I know, the initial multilingual datasets from the early days of deep learning where collected from proceedings of European Parliament, so it’s possible that the model has been trained on similar data. Whisper understands different numbers such as 2475, eleven or three.
Source: https://www.youtube.com/watch?v=DB87Zw7exwQ
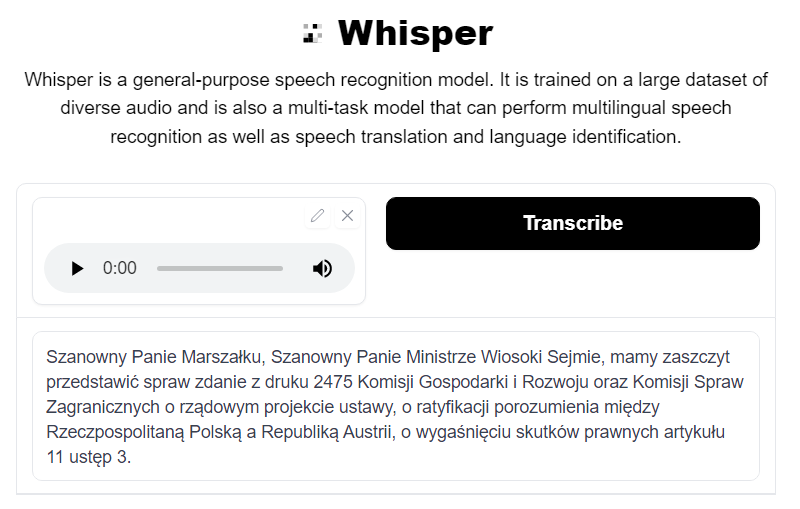
Slang
Input/ground truth 🇵🇱 :
“Witam, chciałbym podzielić się z wami sposobem wysokiego zarobku. Nie jest to żadna ściema! Wszystko co musicie zrobić, to lajkować moje posty na fejsie. Jak uzbieramy dziesięć tysięcy lajków, to zrobimy melanż nad Wisłą dla wszystkich, którzy przyjdą”
English translation 🇬🇧:
“Hello, I would like to share with you how to earn a lot of money. This is not a scam! All you have to do is like my Facebook posts. If we get ten thousand likes, we will make a party at the Vistula river, open for everyone”
Whisper 🗣:
“Witam. Chciałem podzielić się z wami sposobem wysokiego zarobku. Nie jest to żadna ściema. Wszystko, co musicie zrobić, to likować moje posty na fejsie. Jak uzbieramy 10 tysięcy lajków, to zrobimy melanż nad Wisłą na wszystkich, którzy przyjdą.”
Interestingly, the model performed best with informal language. There are practically no mistakes, it only wrote “lajkować” (which means “to like”, specifically in the context of clicking a button on facebook or instagram) as “likować” (which is closer to the original word in English) and I’m pretty sure the preference between the two depends on the person.
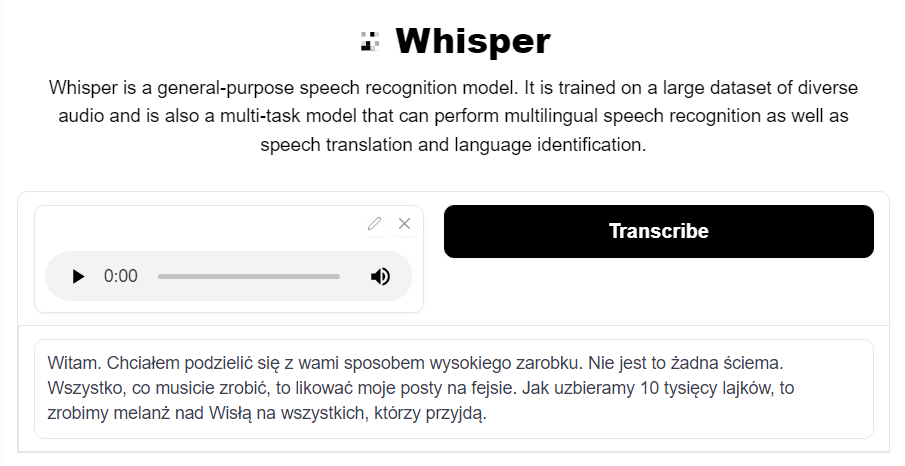
Medical textbook
Input/ground truth 🇵🇱 :
“Więzadła żółte są ważnym elementem strukturalnym kanału kręgowego. W przypadkach zmian zwyrodnieniowych kręgosłupa mogą one ulec hipertrofii, często powiązanej z przerostem oraz zmianami artretycznymi stawów międzykręgowych.”
English translation 🇬🇧:
“Yellow ligaments are an important structural element of the spinal canal. In cases of degenerative changes in the spine, they may become hypertrophied, often associated with hypertrophy and arthritic changes in the intervertebral joints.”
Whisper 🗣:
“Więc odła żółte są ważnym elementem strukturalnym kanału kręgowego. W przypadku jak zmian z wyrodnieniowych kręgosłupa mogą one ulec hipertrofi, często powiązanej z przerostem oraz zmianami artytetycznymi stawów międzykręgowych.”
I expected this topic to be the most challenging since it’s full of domain-specific terms. The results follow these expectations, but I’m impressed by some very rare words that were correctly understood such as “hipertrofii” (EN: “hypertrophy”) and “międzykręgowych” (EN: “intervertebral”).
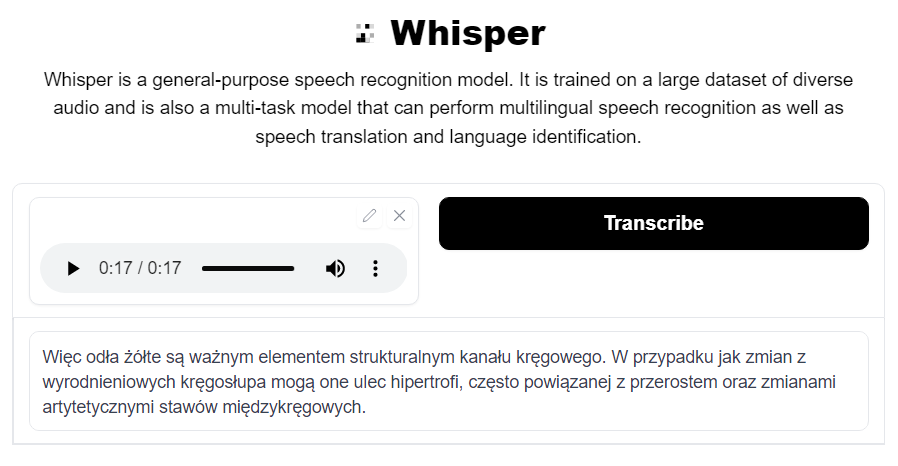
Summary
- Considering that Whisper was trained on tens of languages, its zero-shot performance in Polish is impressive
- Unexpectedly, regardless of the domain, about three words are incorrectly transcribed in every example
- The least number of errors is obtained in the example containing informal language which might happen because the training data was scraped from the internet that contains texts with a wide range of level of formality
- In most of the errors, the model outputs non-existent words or phrases which sound very similar to the input. This probably is the result of using a subword tokenizer (BPE). To mitigate this specific problem, using a word tokenizer would help, but it has been shown over years that the overall performance would probably decrease.
Try it yourself: https://huggingface.co/spaces/openai/whisper